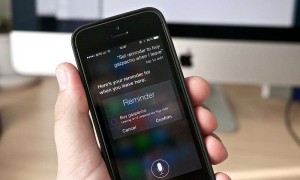
At Apple’s recent World Wide Developer Conference, one of the tent-pole items was the inclusion of additional features for intelligent voice recognition by its personal assistant app Siri in its most recent update to its mobile operating system iOS 9.
Now, instead of asking Siri to “remind me about Kevin’s birthday tomorrow”, you can rely on context and just ask Siri to “remind me of this” while viewing the Facebook event for the birthday. It will know what you mean.
Technology like this has also existed in Google devices for a little while now – thanks to OK Google – bringing us ever closer to context-aware voice recognition.
But how does it all work? Why is context so important and how does it tie in with voice recognition?
To answer that question, it’s worthwhile looking back at how voice recognition works and how it relates to another important area, natural language processing.
A brief history of voice recognition
Voice recognition has been in the public consciousness for a long time. Rather than tapping on a keyboard, wouldn’t it be nice to speak to a computer in natural language and have it understand everything you say?
Ever since Captain Kirk’s conversation with the computer aboard the USS Enterprise in the original Star Trek series in the 1960s (and Scotty’s failed attempt to talk to a 20th-century computer in one of the later Original Series movies) we’ve dreamed about how this might work.
Even movies set in more recent times have flirted with the idea of better voice recognition. The technology-focused Sneakers from 1992 features Robert Redford painfully collecting snippets of an executive’s voice and playing them back with a tape recorder into a computer to gain voice access to the system.
But the simplicity of the science-fiction depictions belies a complexity in the process of voice-recognition technology. Before a computer can even understand what you mean, it needs to be able to understand what you said.
This involves a complex process that includes audio sampling, feature extraction and then actual speech recognition to recognise individual sounds and convert them to text.
Researchers have been working on this technology for many years. They have developed techniques that extract features in a similar way to the human ear and recognise them as phonemes and sounds that human beings make as part of their speech. This involves the use of artificial neural networks, hidden Markov models and other ideas that are all part of the broad field of artificial intelligence.
Through these models, speech-recognition rates have improved. Error rates of less than 8% were reported this year by Google.
But even with these advancements, auditory recognition is only half the battle. Once a computer has gone through this process, it only has the text that replicates what you said. But you could have said anything at all.
The next step is natural language processing.
Did you get the gist?
Once a machine has converted what you say into text, it then has to understand what you’ve actually said. This process is called “natural language processing”. This is arguably more difficult than the process of voice recognition, because the human language is full of context and semantics that make the process of natural language recognition difficult.
Anybody who has used earlier voice-recognition systems can testify as to how difficult this can be. Early systems had a very limited vocabulary and you were required to say commands in just the right way to ensure that the computer understood them.
This was true not only for voice-recognition systems, but even textual input systems, where the order of the words and the inclusion of certain words made a large difference to how the system processed the command. This was because early language-processing systems used hard rules and decision trees to interpret commands, so any deviation from these commands caused problems.
Newer systems, however, use machine-learning algorithms similar to the hidden Markov models used in speech recognition to build a vocabulary. These systems still need to be taught, but they are able to make softer decisions based on weightings of the individual words used. This allows for more flexible queries, where the language used can be changed but the content of the query can remain the same.
This is why it’s possible to ask Siri either to “schedule a calendar appointment for 9am to pick up my dry-cleaning” or “enter pick up my dry-cleaning in my calendar for 9am” and get the same result.
But how do you deal with different voices?
Despite these advancements there are still challenges in this space. In the field of voice recognition, accents and pronunciation can still cause problems.
Because of the way the systems work, different pronunciation of phonemes can cause the system to not recognise what you’ve said. This is especially true when the phonemes in a word seem (to non-locals) to bear no relation to the way it is pronounced, such as the British cities of “Leicester” or “Glasgow”.
Even Australian cities such as “Melbourne” seem to trip up some Americans. While to an Australian the pronunciation of Melbourne is very obvious, the different way that phonemes are used in America means that they often pronounce it wrong (to parochial ears).
Anybody who has heard a GPS system mispronounce Ipswich as “eyp-swich” knows this also goes both ways. The only way around this is to train the system in the different ways words are pronounced. But with the variation in accents (and even pronunciation within accents) this can be quite a large and complex process.
On the language-processing side, the issue is predominantly one of context. The example given in the opening provides an example of the state of the art in contextual language processing. But all you need to do is pay attention to a conversation for a few minutes to realise how much we change the way we speak to give machines extra context.
For instance, how often do you ask somebody:
Did you get my e-mail?
But what you actually mean is:
Did you get my e-mail? If you did, have you read it and can you please provide a reply as response to this question?
Things get even more complicated when you want to engage in a conversation with a machine, asking an initial question and the follow-up questions, such as “What is Martin’s number?”, followed by “Call him” or “Text him”.
Machines are improving when it comes to understanding context, but they still have a way to go!
Automatic translation
So, we have made great progress in a lot of different areas to get to this point. But there are still challenges ahead in accent recognition, implications in language, and context in conversations. This means it might still be a while before we have those computers from Star Trek interpreting everything we say.
But rest assured. We are slowly getting closer, with recent advancements from Microsoft in automatic translation showing that, if we get it right, the result can be very cool.
Google has recently revealed technology that uses a combination of image or voice recognition, natural language processing and the camera on your smartphone to automatically translate signs and short conversations from one language to another for you. It will even try to match the font so that the sign looks the same, but in English!
So no longer do you need to ponder over a menu written in Italian, or wonder how to order from a waiter who doesn’t speak English, Google has you covered. Not quite the USS Enterprise, but certainly closer!
Michael Cowling is Senior Lecturer & Discipline Leader, Mobile Computing & Applications at Central Queensland University.
References:http://phys.org/